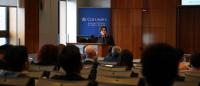
Data Science Summit Looks to the Future of Public Health
Over the past year, AI tools like ChatGPT and DALL·E have made headlines for their ability to generate text and images in powerful new ways. Meanwhile, public health data scientists are increasingly harnessing advanced analytic methods like AI, with major implications for the future of the field.
A daylong Data Science for Public Health Summit at Columbia University Mailman School of Public Health examined the critical and growing importance of emerging analytic tools, from machine learning and natural language processing to network science, dynamic modeling, and data visualization. Participants included faculty from across Columbia Irving Medical Center and the larger university, including representatives from Columbia’s Data Science Institute.
The goal of the summit was to assess the field of data science for health, including both its current status and future trends, alongside a thorough assessment of how Columbia could structure its research and training activities to be at the vanguard of the discipline.
Guest speakers and panel discussions covered topics, including approaches to training the next generation in data science in public health, ethical issues in data science, and practical considerations around the infrastructure for data science research. Like the first Data Science for Public Health Summit in early 2020, the 2023 summit was organized and moderated by Gary Miller, Vice Dean for Research Strategy and Innovation and professor of Environmental Health Sciences—together with Kiros Berhane, chair of Biostatistics, and Jeff Goldsmith, associate professor of Biostatistics.
In opening remarks, Dean Linda P. Fried said data science will play a key role in addressing critical public health challenges in the coming years. “The complexity of the challenges we’re confronted with demand we look at the methods and the capabilities we as a field bring to the future of health,” she said. “Schools and programs of public health have to become facile in the wide range of tools and techniques that fall under the data sciences.”
The Dean observed that data science has the potential for both good and bad, with the latter including the potential to exacerbate health disparities. As a field, she said, public health needs to create training and research programs that prevent these missteps and create safeguards for every sector to use.
Jeannette M. Wing, executive vice president for research at Columbia University and formerly the director of the Data Science Institute, said the rapidly growing collection of data, combined with our ability to analyze it is putting new demands on data science infrastructure. Wing said her “big goal” is to centralize high-performance computing infrastructure at the university, providing the computing power and storage to support data science, including “high-end AI applications, scientific computing, and gobs and gobs of data.” She solicited input on this plan, encouraging faculty to dream big about what they might do with the right tools and infrastructure.
Xihong Lin, a biostatistician on the faculty of Harvard T. H. Chan School of Public Health, delivered a keynote address that reflected on lessons learned from conducting and communicating research on the COVID-19 pandemic. Among these lessons was the need for data scientists to “step in early and learn on the fly.” Pre-print publications and real-time dashboards provided actionable information in a fast-moving situation. Another important lesson: media training to help scientists communicate with journalists.
An Eye to Ethics
Summit speakers and panelists shined a light on the need for ethical guidelines in data science. As one example of an ethical lapse, an algorithm used by hospitals was found to discriminate against Black people by not referring them to care in the same way it did for White people.
In a distinguished lecture on the eve of the summit, Sherri Rose, a professor of health policy and co-director of the Health Policy Data Science Lab at Stanford University, said ethics, fairness, and health equity should all be considerations for AI tools, all of which should be individually assessed for whether or not they exacerbate harms to minoritized groups. Rose went on to describe her own research which uses AI to reduce inequities in health insurers’ use of risk adjustment formulas. Done right, Rose said AI “could more frequently be contributing to improving health equity rather than harming it.”
In a related panel discussion, Gary Miller said the Columbia Mailman School can take a lead in teaching students in ethical data science before they take jobs in health care technology. “We can start by infusing our values in the people trained at the university,” he said.