Faculty: Yifei Sun
Yifei Sun, PhD
Assistant Professor of Biostatistics
My research merges survival analysis, semiparametric methods, and machine learning techniques, with application to complex time-to-event data commonly seen in longitudinal studies and electronic health records. The Sanford-Bolton Award allows me to devote more time to methodological development and explore more interesting research topics.
Many of my recent projects involve the interplay between longitudinal and survival data. In many studies, repeated measurements of health processes (e.g., biomarkers) are collected prior to the occurrence of an event (e.g., disease progression, death). The complex data structure poses challenges in methodological development; on the other hand, it also brings opportunities to track the evolution of disease and improve risk management.
For example, we can incorporate time-varying patient information to improve event risk prediction. To exploit the growing amount of predictor information over time, we developed a unified framework for landmark prediction using survival tree ensembles, where an updated prediction can be performed when new information becomes available. Our methods allow the landmark times to be subject-specific and triggered by an intermediate clinical event. The methods can also be extended to conduct dynamic risk prediction of multiple events, as many chronic diseases are characterized by a series of nonfatal events.
Figure: An illustration of dynamic risk prediction. Curves on the left show the repeated measurements before the landmark time for six subjects. Curves on the right show the survival predictions over the interval of interests for the corresponding groups. The upper panel is for prediction at landmark age 7. With the additional information between age 7 and 12, we can update the prediction at age 12 (lower panel).
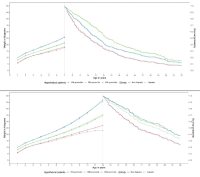
As another example, the longitudinal and time-to-event data also allows us to uncover the temporal changes of biomarkers prior to disease onset, and this offers insights into early diagnosis and natural disease history. Existing methods often provide an overall biomarker trajectory without explicitly accounting for the impact of the time of disease onset on the trajectory shape. However, in some applications, subjects with early disease onset may have different trajectories, such as a faster rate of decline, so a more accurate characterization of trajectories should account for the event time. We are now developing semiparametric models to capture the trends of biomarkers in multiple time scales.