Daniel Malinsky, PhD
- Assistant Professor of Biostatistics
On the web
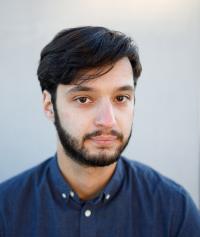
Overview
Daniel Malinsky's methodological research focuses mostly on causal inference: developing statistical methods and machine learning tools to support inference about treatment effects, interventions, and policies. Current research topics include graphical structure learning (a.k.a. causal discovery or causal model selection), semiparametric inference, time series analysis, and missing data. Application areas of particular interest include environmental determinants of health and health disparities. Dr. Malinsky also studies algorithmic fairness: understanding and counteracting the biases introduced by data science tools deployed in socially-impactful settings. Finally, Dr. Malinsky has interests in the philosophy of science and the foundations of statistics.
Before joining the Mailman School of Public Health, Dr. Malinsky was a postdoctoral fellow at Johns Hopkins University.
Academic Appointments
- Assistant Professor of Biostatistics
Credentials & Experience
Education & Training
- BA, 2011 Columbia University
- MS, 2015 Carnegie Mellon University
- PhD, 2017 Carnegie Mellon University
Research
Research Interests
- Biostatistical Methods
Selected Publications
D. Malinsky, I. Shpitser, and E. J. Tchetgen Tchetgen (2020+) "Semiparametric Inference for Non-monotone Missing-Not-at-Random Data: the No Self-Censoring Model." Journal of the American Statistical Association.
R. Bhattacharya, D. Malinsky, and I. Shpitser (2019) “Causal Inference Under Interference and Network Uncertainty.” In Proceedings of the 35th Conference on Uncertainty in Artificial Intelligence (UAI).
R. Nabi, D. Malinsky, and I. Shpitser (2019) “Learning Optimal Fair Policies.” In Proceedings of the 36th International Conference on Machine Learning (ICML).
D. Malinsky, I. Shpitser, and T. S. Richardson (2019) “A Potential Outcomes Calculus for Identifying Conditional Path-Specific Effects.” In Proceedings of the 22nd International Conference on Artificial Intelligence and Statistics (AISTATS).
D. Malinsky and P. Spirtes (2019) “Learning the Structure of a Nonstationary Vector Autoregression.” In Proceedings of the 22nd International Conference on Artificial Intelligence and Statistics (AISTATS).
S. W. Mogensen, D. Malinsky, and N. R. Hansen (2018) “Causal Learning for Partially Observed Stochastic Dynamical Systems.” In Proceedings of the 34th Conference on Uncertainty in Artificial Intelligence (UAI).